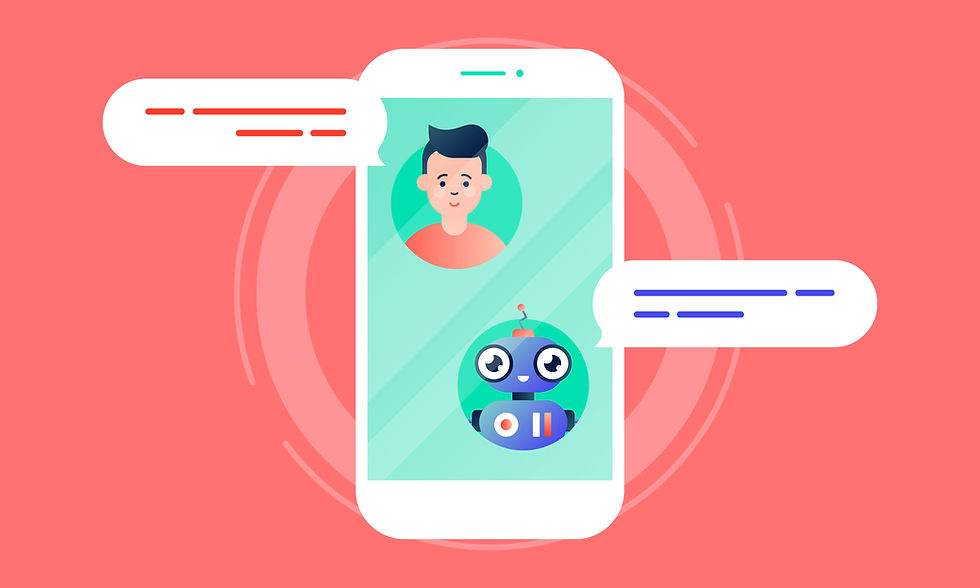
Machine Learning (ML) has been applied in Robo Advisory to improve the advisory quality, this article discusses areas of Robo Advisory that ML could be applied to.
Robo advisory provides investment advisory to banks’ clients or Relationship Managers (RM) through a digital platform with minimal human intervention. More and more banks and clients are turning to Robo Advisory because of its user friendliness, convenience of anytime anywhere and cost competitiveness. Basically, Robo advisory is a process of evaluation of client investment profile, portfolio/product profile and a matching process of these profiles. Today's investment advisories are using simple algorithms. For instance, matching clients with low investment risk with low product risk rating portfolio. In order to turn the advisory to be sophisticated, several advanced research, especially in the area of ML, is being conducted. Below are three areas where ML could be applied to. Portfolio Profile Today’s portfolio profile update is too slow in the fast changing financial market. New ML technology is applied to analyze the market data and update the portfolio profile on a real time basis to help clients seize every investment opportunity. A ML model could be built for the relationship of price volatility of stocks in the portfolio and the portfolio risk metrics. So the model could dynamically adjust the risk metrics, e.g. risk rating, of the portfolio. Client Investment Profile Nowadays client investment profiles only contain static data, e.g. year of experience in certain products. They do not include the client investment behaviour which is continuously evolving and critical to the advisory process. ML could learn the client investment behaviour, and its change, through the scanning of investment decisions. For example, a shifting of investment decisions from stock to fund may indicate a change of client investment appetite. The update of the client behaviour will not be applied automatically because of the legal obligation from the banks. However, RM will be alerted for the client investment behaviour change and confirm with the clients for their new preference. Hence to make the robo advisory giving more suitable investment advice. Advisory Process Currently the advisory process is a hardcoded matching process. The process itself is not self-evolving through the learning of historical investment transactions. ML technology, such as Deep Learning, could be applied to make the advisory process more intelligent. Historical and on-going profile and transactions could be used as training data for continuous training of the advisory process. For example, the initial advisory may give investment advice of selling Microsoft and buying Amazon. After learning the investment transactions for one month, it may make a self adjustment. So under the same circumstance, it will give a different investment advice. Such self adjustment is the most powerful part of Machine Learning and unmatched by the traditional advisory process. Thank you for reading the article. Here are some other articles from FinTech Insights:
For the details of the article, please contact us. If you want to receive more information about finance and technology, please follow our LinkedIn or subscribe to the “FinTech Insights”.

Axisoft is a Top-Notch Financial Technology Provider in Hong Kong, Singapore and China. Since 1998, we have been helping banks implement global banking solutions.
Copyright © 2022 Axisoft. All Rights Reserved